Frameworks for Gauging AI Opportunities in Professional Services
Generative AI doesn't spell doom for professional services. Discover how to assess its impact with strategic frameworks that help owners and investors measure AI effects, understand competitive pressures, and plan responses to drive efficiency and growth.
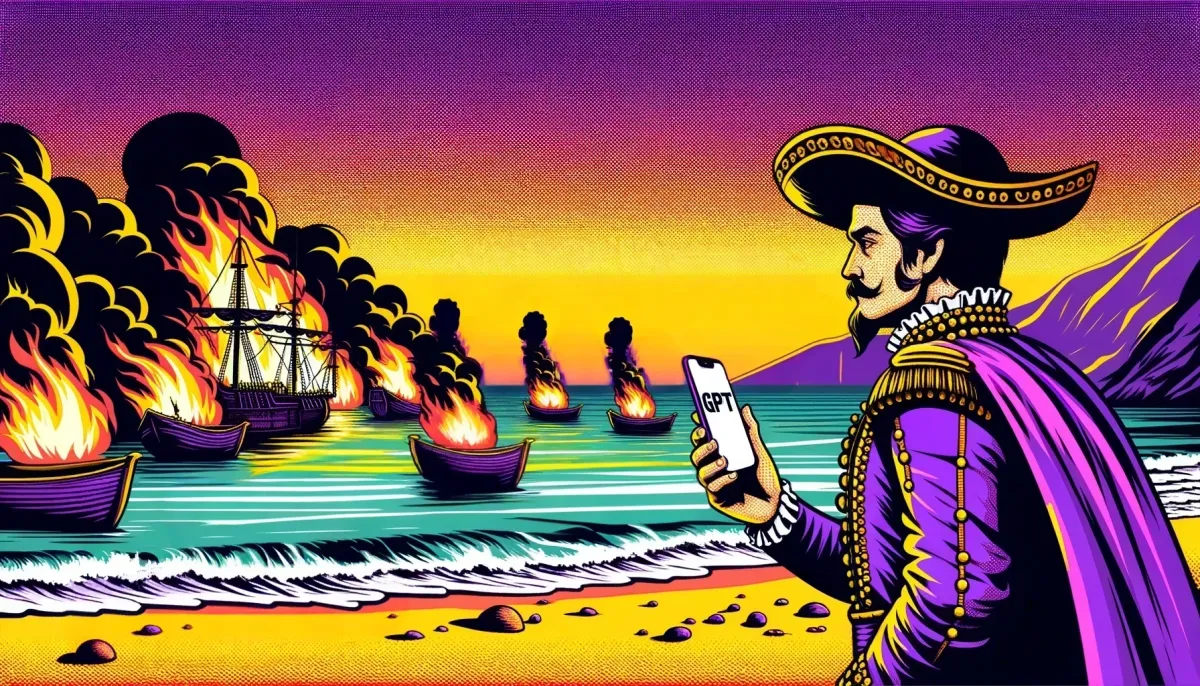
Since the public release of generative AI models in late 2022, professional services has been the most common industry I’ve seen investors raise questions about its potential impact. The sector is a popular thesis for private equity, due to potential roll-up synergies and a pliable business model favouring variable over fixed costs.
AI raises concerns about its ramifications on human capital, competition from self-service solutions, and implications for time-and-materials-based pricing. Consequently, many businesses are focused on devising AI strategies. Responses range from investors interested only in AI's high-level impact to emphasise its narrative trajectory, to those rushing into developing unvalidated, externally-facing AI products, often overlooking the potentially greater benefits of internal operational efficiency.
As a balanced approach between AI theatre and gambling, this article provides frameworks for assessing its impact on professional services from both internal and external perspectives, and for structuring a strategic response.
After numerous engagements across various sectors, I am optimistic about AI being a net benefit; it offers an opportunity to redefine linear structures within professional services firms. Viewing AI as an internal enabler rather than a client-facing tool may facilitate the breakdown of silos and the development of cross-functional “full-stack” staff profiles.
How to Assess AI Impact on a Business
The optimal approach to evaluating AI’s effect on a business is to blend the creative, unconstrained aspects of futurology and strategy landscaping with the pragmatic aspects of process mapping.
Anchoring work around the internal value chain of the firm is an effective way to contextualise impacts and identify solutions. A bottom-up assessment of workflows within the value chain can be used to heatmap areas ripe for automation:
- Verbs: Is the task based around text, image, audio, coding, etc? I.e., generative activities
- Outcomes: What does the task achieve? E.g., optimisation, decision-making, productivity or new business generation
- Idiosyncrasies: Is the process rote or creative? Can we free up time to focus on more exciting tasks?
- Bottlenecks: What is the seniority of decision-making, the difficulty of the task, and the reliance on third-party tools?
The granular process work can be combined with top-down competitive market mapping: identifying commercial products replicating tasks and analysing competitors' moves. Examining competitors also addresses business leaders' anxieties and game theory aspects about AI; it is not just about responding to AI but ensuring they are not the last to adopt it.
1. Consider the External Market’s AI Pressure Points
The most MECE (Mutually Exclusive, Collectively Exhaustive) way of considering AI’s external impact from direct and adjacent competitors is through AI’s commercial “Pressure Points”. The fundamentals of how AI will impact companies will distil into behaviours and enablers, through which competitors exert pressure by harnessing AI to gain advantages on a relative or absolute basis.
- Price: Will automation provide competitors or new entrants with a cost advantage that is passed through to pricing?
- Time: Will the adoption of generative AI in processes speed up competitors through automated solutions or more efficient delivery?
- Scale: Will AI bring marginal cost and capacity gains?
- Nuance: Will AI bring differentiation through originality of insights?
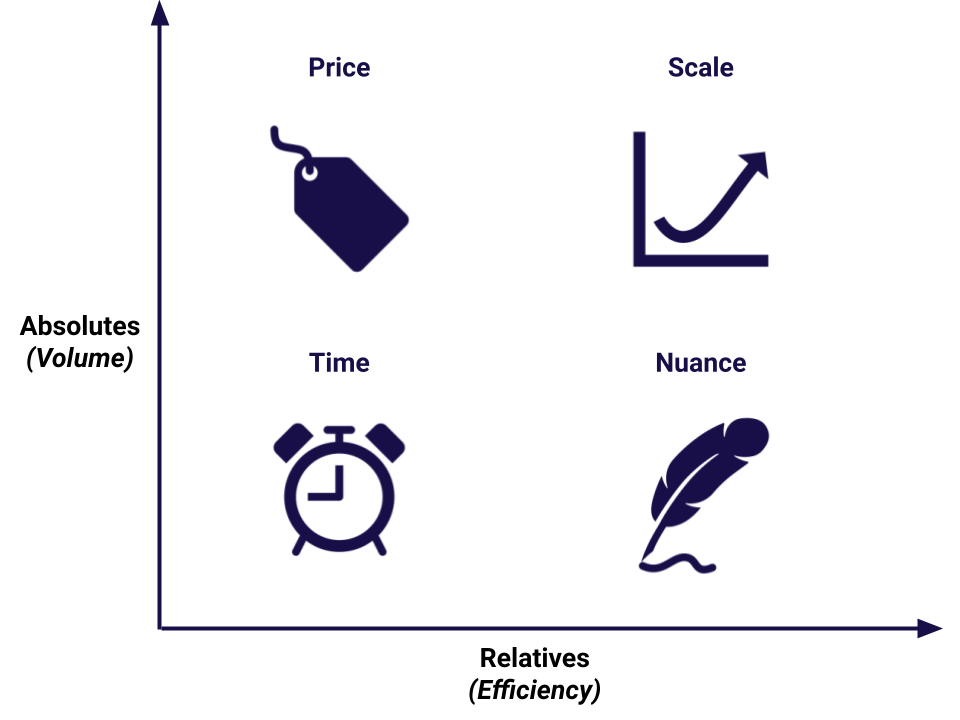
Identifying where pressure points are likely to arise can serve as a call to action for channelling broader strategies within the firm. For example, price will be an area for opportunistic competition from new entrants or incumbents seeking quick gains, but pursuing it risks brand cannibalisation. Preparing for a price war can help brands focus on aspects like “organic work” eschewing AI or quality versus quantity of clients served.
2. Opportunities to Simplify the Value Chain
AI has the potential to streamline complex value chains and throughputs. There are abstract parallels between the stages of professional services firms and traditional factory production lines. The production of intangible outputs, managed by junior staff in specific roles and ratified by a partner, can lead to bureaucratic hand-offs and bloated structures. The use of generative AI tools, such as retrieval-augmented generation (e.g., accessing internal documents) and process automation (e.g., image generation), can help corporate structures evolve into more distinct outcomes-based roles.
- Business Development: Decoupling revenue and delivery responsibilities and empowering front-line staff to build tactical sales relationships.
- Production: Full-stack consultants taking work from sale to final delivery, cross-discipline.
- Operational: OPEX-level staff focused on improving margins and cost control.
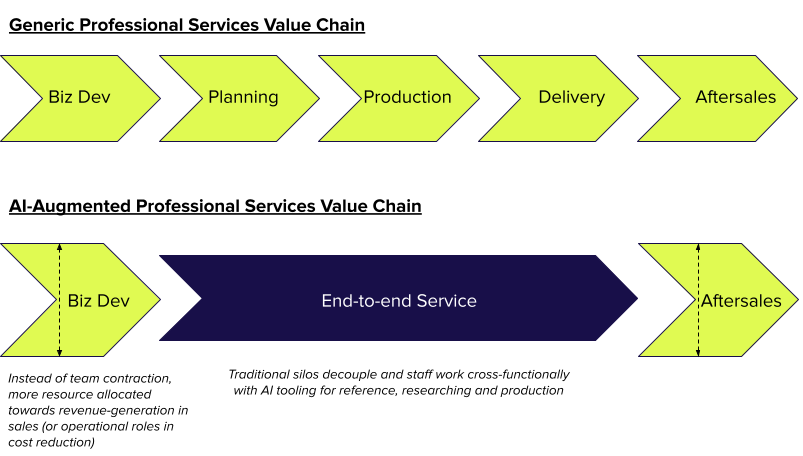
The vision of staff acting as conductors of work while using an array of AI tools may sound dystopian. However, at its most nascent and plausible stage, retrieval tooling allows staff to quickly reference and learn from past work using more intuitive contextual queries to search the firm’s collective knowledge base. This is the first step towards compressing workflow steps and fostering an agile worker mentality capable of handling end-to-end delivery post-sale.
3. Is AI Competitive Advantage Feasible?
A key distinction in the AI world is between being a leaseholder and a freeholder. There are several LLMs, commercial (e.g., OpenAI’s GPT) or open-source (e.g., LLaMA), competing for prominence. Over time, their performance will invariably converge, resulting in a utility-like market, with compute rented out by the traditional cloud infrastructure providers.
Subsequently, merely deploying a popular LLM will not be a source of long-term competitive advantage. Over time, insights and efficiencies from early adopters' LLM usage will diminish and become the new standard (hence the "Nuance" pressure point), akin to how Google search became the baseline for discovery.
Becoming a freeholder involves establishing a differentiated advantage through having unique data to train private LLM instances and/or network effects to train a model on an engaged user base. For example, Grammarly is seen as a business model that ChatGPT’s functionality potentially makes obsolete. Yet, if over the years Grammarly has trained and refined a private instance of an LLM with its user data, its proposition may yet remain differentiated.
The advantages of being a leaseholder are financial and operational; you can bootstrap off the advances of others and avoid significant sunk cost investments. The corollary though is a commercial existence contingent on an external platform’s benevolence. Many businesses putting UI wrappers over ChatGPT, Claude, etc., may have a false sense of security about their defensibility and differentiation. Such ventures will be commodity offers without any competitive advantage unless they acquire unique data and network effect assets.
4. Build Consensus on the Strategic Response Appetite
When plotting an AI programme, believe that less can be more. A simple rollout of a third-party productivity tool may be more accretive than an overly complex, exorbitantly priced solution responding to non-existent threats. Considering the external market conditions, companies should assess their appetite for AI-driven transformation, mapped along a strategic philosophy (buy/rent vs. build) versus pace axis:
- Status Quo: Incremental internal AI adoption, assuming current business model prevails.
- Hedged: Investing in or incubating new practices in isolation from BAU.
- Evolution: Deeper AI adoption into workflows, assuming notable change to business model.
- Burn the Boats: Comprehensive change under the premise AI will disrupt the entire paradigm.
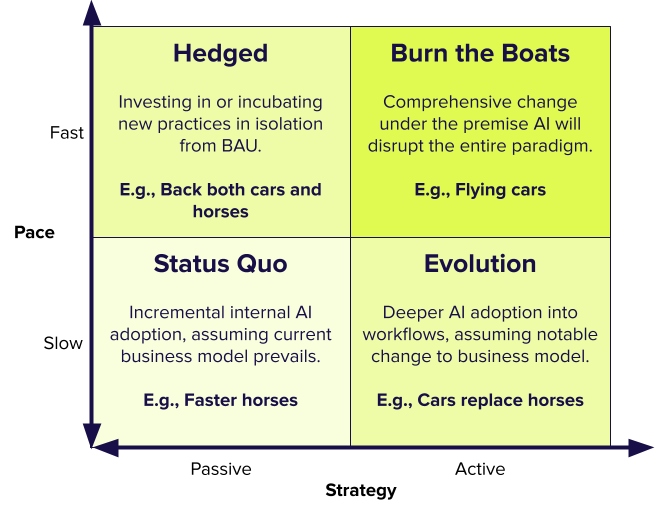
Aligning internal appetite with external conditions ensures a measured response. For example, while a coffee chain owner may want to "burn the boats", their industry's benign outlook negates the need for drastic change.
Avoid AI Theatre
Investors are right to exercise caution when mulling over AI experimentation projects. As with other trends (E.g., Web3, Metaverse, etc.), its zeitgeist status will ebb and flow, and the market is rife with dubious solutions promising AI-enriched benefits. For some, the AI theatre toe dip is all that’s required, as the goal is to pique the next investor’s interest (and pockets) with the AI transformation narrative.
However, to unlock AI’s full potential in professional services, decision-makers must move beyond lip service and embrace the analytical work and bravery to enact change. Using AI to remove silos and fiefdoms, and moving toward simpler models with AI-augmented cross-functional talent, will improve client outcomes and retention, positioning the firm in a more operationally flexible manner.
My view is that the fear of AI aggressively disrupting B2B services is a fallacy. Industries evolve: marketing, advertising, and accountancy adapted to new technologies, leveraging them to grow and respond to changing client needs. Clients will still have nuanced issues that self-service AI cannot solve; the solution type may change (e.g., automated marketing copy), but the customer intent will prevail (e.g., how to enter a new market). AI tools will serve more as an accomplice than an adversary, helping elevate service levels. Did Excel render accountants irrelevant?